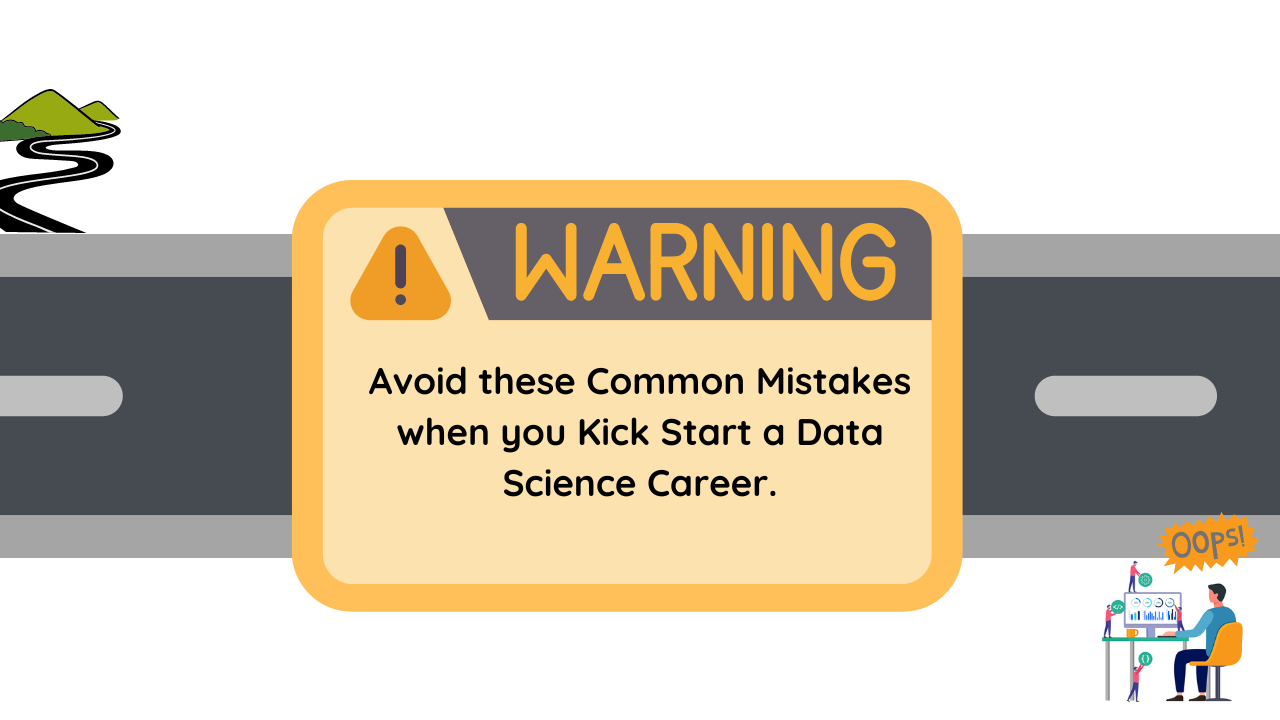
8 Mistakes To Avoid When You Begin a Data Science Career
Sep 21, 2024 7 Min Read 1770 Views
(Last Updated)
With leading modern-day companies relying on Data Science professionals to make more informed decisions and bring in better solutions, the field of data science is constantly evolving. We agree that the demand for data scientists is steadily increasing. On the other hand, data science is hands down one of the competitive fields where you need to be a skillful professional with appropriate expertise to sustain your stand in the field.
If you’re a data science aspirant, practice to stay flawless from the beginning of your career. This blog highlights the beginner mistakes you should avoid when you start a Data Science career. Let’s dive in!
Table of contents
- Common Mistakes to Avoid When Starting Your Data Science Career
- Not Learning the Fundamentals
- Lack of Practical Experience & Skills
- Not Creating an Intriguing Portfolio
- Not keeping Up with Trends & the Latest Technologies
- Lack of Commitment, Consistency, and Planning
- Underestimating the Power of Domain Knowledge
- Lack of Communication
- Losing Time & Effort in Choosing the Right learning platform
- Wrapping up
- FAQs
- How do I start a data science career for beginners?
- Can a beginner do data science?
- Is data science hard for non-programmers?
- How to start a career in data science with no experience?
- What is the fastest way to learn data science?
Common Mistakes to Avoid When Starting Your Data Science Career
You will save a lot of time, energy, and money if you are careful not to commit these simple mistakes, such as not prioritizing the knowledge of fundamentals, lack of practical knowledge, not creating a sound portfolio, not staying updated with technologies, lack of commitment, consistency, planning, neglecting domain knowledge, and so on…
This blog breaks down 8 common mistakes that a beginner in data science is most likely to commit when setting up a career in Data Science, the common pitfalls, and how to avoid them.
The entire list includes mistakes that come your way during these four instances: while learning when applying for a job, during job interviews, and after joining the job. Read the complete article to ensure that you don’t fall prey to these common pitfalls during your trial and, thus, build a rock-solid Data Science career.
1. Not Learning the Fundamentals
Data Science is a buzzword in the technology-driven world today. But it is so true to state that only a few people understand Data Science in its true sense. This is because professionals and students who try to enter the field blindly rely on trends and neglect the fundamental concepts of Data Science.
Many Data science aspirants believe that having a list of skills mentioned in the resume will help land a high-paying job which isn’t true. Learn the fundamentals first to build a strong foundation and then hop on to niche topics in Data science.
Master the fundamentals of data science like mathematics, programming, and statistics before you start to work on any projects. These basics will lay a firm foundation for your solid data science skills and career. Make sure that you don’t skip the key concepts and algorithms. Not having a solid foundation in machine learning, data visualization, probability, and statistics will affect your career as you think about growth.
For example, if you’re reading the topics of machine learning, try to understand how they work, respond to specific types of data, and how their hyper-parameters affect the training. Revisit the basics when needed. Always remember that the fundamentals will keep you alive in the field.
Before we move into the next section, ensure you have a good grip on data science essentials like Python, MongoDB, Pandas, NumPy, Tableau & PowerBI Data Methods. If you are looking for a detailed course on Data Science, you can join GUVI’s Data Science Course with Placement Assistance. You’ll also learn about the trending tools and technologies and work on some real-time projects.
Additionally, if you want to explore Python through a self-paced course, try GUVI’s Python course.
2. Lack of Practical Experience & Skills
Don’t depend too much on theoretical concepts. Of course, they are significant but don’t waste all your time reading about the terminologies, methods, etc., The theory-savvy approach will lead to procrastination. Eventually, you’ll lose interest too.
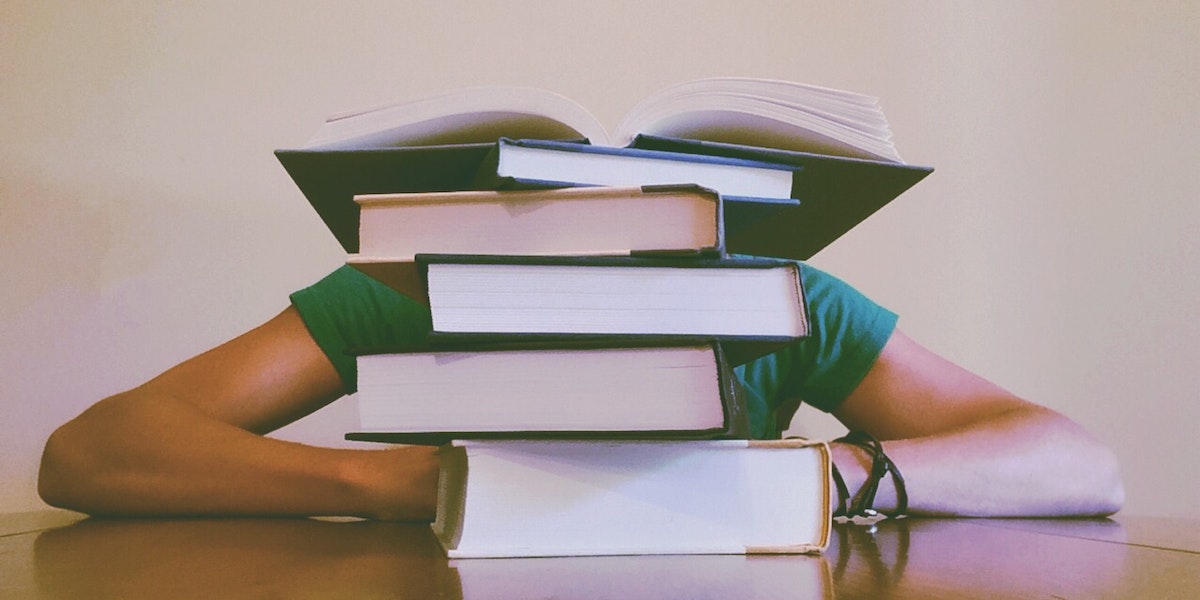
Add practical sessions to your learning plan. Get some technical know-how to solve real-life business problems with your Data Science skills. Learning data science is a process of trial and error. Try the techniques, store your work, revisit the projects, learn from the failures, document quick fixes, and so on…
By practical learning, we don’t mean you should hop into the data and start to code right away. As you progress, spend adequate time researching and understanding the business problems. Create a project plan and identify the proper method to solve the business problem. Try to analyze the project, map the available data sources, write down the success metrics, and learn how a realistic roadmap is applied to meet time constraints.
One of the most significant responsibilities of data scientists includes skillfully expressing and communicating complicated statistical insights to the stakeholders and helping them make more informed business decisions.
A data scientist should be a top-notch professional with technical, non-technical, and interpersonal skills. Laying the groundwork, learning the know-how, and adapting to these skills are very important. Learn and build your expertise in the most in-demand skills. The rest will fall into place as you progress.
Building your analytical and listening skills and improving your patience will make you a successful data science professional. You’ll also require a good understanding of relational databases, SQL, Excel, Machine learning, and other related tools to withstand your job role.
If you’re stuck about where to start? And how to build proven data science skills? Read this detailed article that explores a profound Data science career path.
3. Not Creating an Intriguing Portfolio
Most people learn the theories of data science concepts and start searching for jobs. It is not how it looks. You’ll need a lot of practice, even if you are so good at coding and algorithms. Start your learning process from scratch. Learn the basic mathematical and programming concepts and understand where it fits in the bigger picture.
Soil your hands on numerous real-life projects. Start with the simple ones and progress to the advanced projects. This practice will give you a vast knowledge of different techniques used so you will learn which ones work for you.
Most importantly, document all your work and sample projects with notes – a list of things that worked out and the things that didn’t. Create a stunning portfolio in GitHub or other sharing sites to exhibit your best work and highlight your data storytelling and visualization skills.
Not creating a portfolio is a serious blunder that data science beginners most likely commit. A portfolio describes your nature of work and gets you a step closer to the job offer than your resume. Stand clear and concise when you brief these projects in the Data Science job interview. Don’t just mention the tools or programming languages you’ve used. Explain how you utilized them and how they impacted your project results.
4. Not keeping Up with Trends & the Latest Technologies
Data Science is a field with a steady spike. It requires a lot of learning. Not keeping up with upcoming trends and the latest technologies can make you fall behind the competition. There are millions of resources on the web to get started with continuous learning.
For instance,
- Read Data science books that best clarify business problems and technical ideas
- Watch Youtube videos that break down technical concepts into simple and understandable bytes.
- Sign up for webinars that give you insights into data science. These short-time live sessions will bring a new perspective to your study.
- Specifically, Participate in the webinars & podcasts that deal with roadmaps, career paths, and scope in the industry are complete career guides. These sessions will talk about all the latest news in the industry.
- Scroll through social networks like Twitter, LinkedIn, Quora, GitHub, and other sites, to understand recent industry updates, trends, and technological advancements.
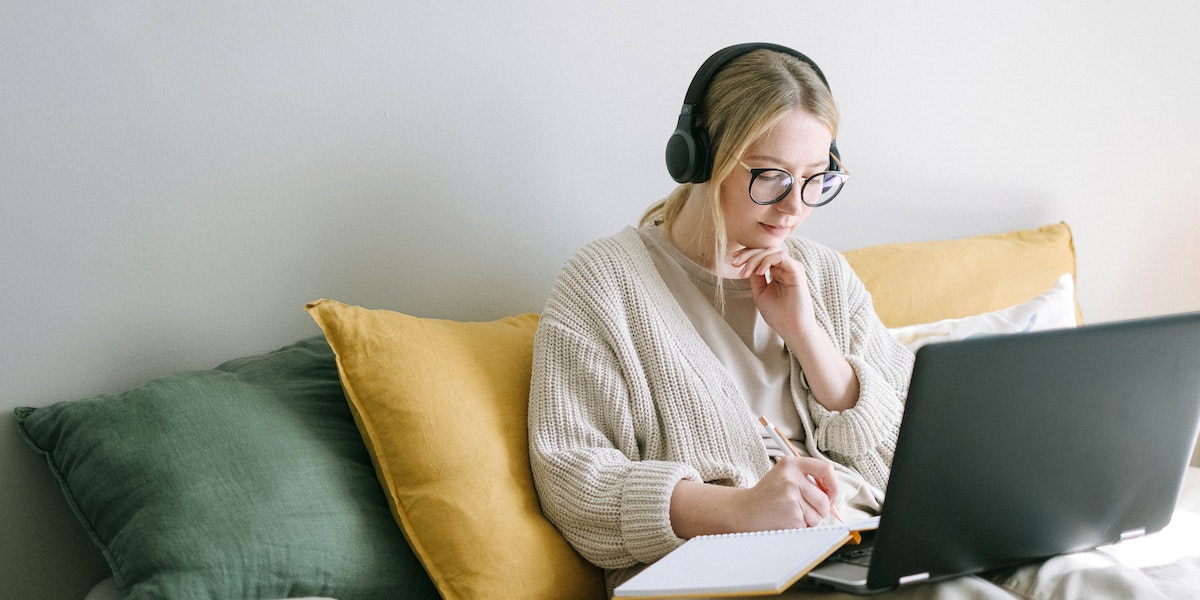
Utilizing these resources in your leisure time will help you stay up-to-date with the latest tools, technologies, and best practices in the field.
5. Lack of Commitment, Consistency, and Planning
Preparing for your Data Science career involves consistent efforts, long-term commitment, and clear-cut planning.
If you spend a few hours on a topic, it doesn’t mean that you’ve reached a saturated level of knowledge. Mastering data science isn’t an overnight process. Work consistently and patiently towards your learning goal. Construct a study plan, customize a strategy, and follow the daily targets.
Document your tasks for the day and revisit the topics whenever needed. The topical knowledge and skills required to become a data scientist don’t come from your extensive learning. For example, your level of problem-solving and analysis might differ from time to time, but with consistent practice, you will develop these skills inevitably.
- Allow yourself some hours a week to improve your existing knowledge. This practice will help you stay updated as well.
- If you’ve signed up for an online course or knowledge-base newsletter or subscribed to any of the resources, ensure you participate in the process consistently. It is natural to feel disconnected from the topic at the beginning. Eventually, you’ll get more enthusiastic about it. So, don’t skip a day.
- Pat yourself when you achieve all milestones from your study plan. Don’t feel demotivated if you ever miss a day or two. Be sure to get back on track sooner with more energy.
6. Underestimating the Power of Domain Knowledge
When you’re a data scientist, you should present the analyzed data to technical and non-technical audiences. You need to sound familiar and insightful for both the crowd. Your domain knowledge will save your day in situations like this. Since you’re solving business problems, your domain knowledge is crucial.
The one solution to overcome this mistake is by researching and learning about the business you’re working with. Reach out to data scientists in your network to know how they overcame this hurdle.
Also, talk to the experts in various fields of business like Tech, HR, finance, Marketing, and sales and find out how their business works. Don’t stress too hard! Domain expertise might also come with experience. So always be open to learning and rejoice in the process.
7. Lack of Communication
Most organizations look for candidates with excellent communication skills to fit their Data Science role because data scientists are entitled to effectively communicate the analyzed data, the solution to the problem, and the possible ways to achieve it to the stakeholders.
To avoid this pitfall, you can practice conveying your data findings to a non-technical audience (probably your non-tech friend). This exercise will help you examine your pain points and work on them.
Another key solution is to join the online community of data science enthusiasts & data science experts. This network will open gates to the Knowledge hub where you share and learn data science.
These networks will actively discuss industry tools, hiring trends, recent advancements, and more. Keeping yourself engaged in such forums is a way of staying on the same page as your fellow data scientists. You can also build networks by participating in webinars, podcasts, boot camps, hackathons, and more.
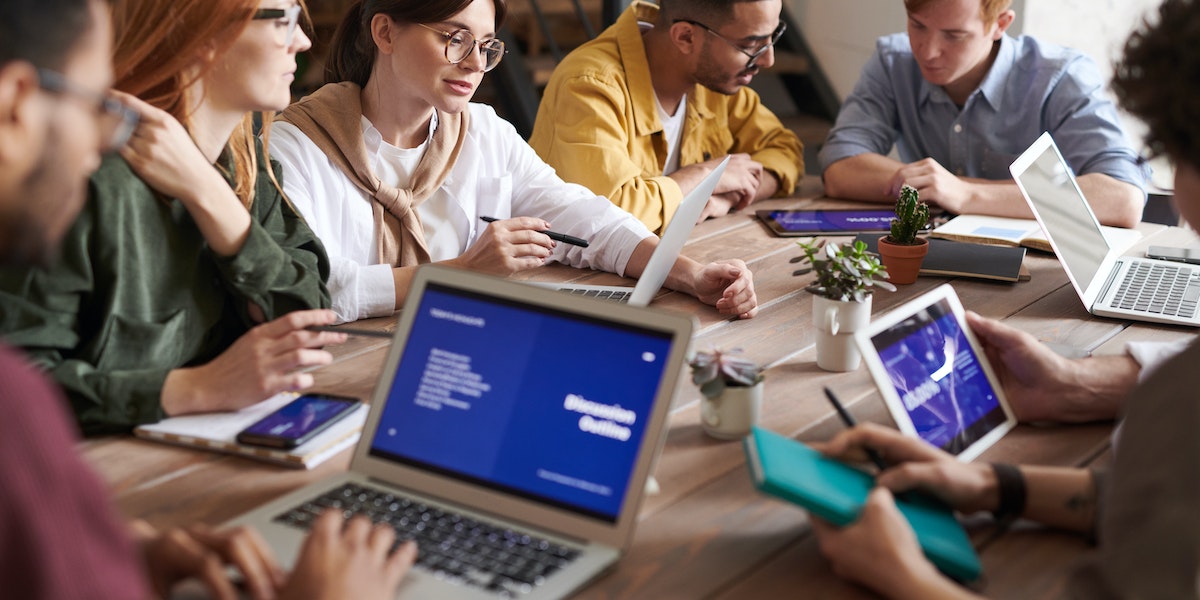
8. Losing Time & Effort in Choosing the Right learning platform
It can be overwhelming to learn all by yourself. So you search for resources online. One of the immediate mistakes here is opting for unstructured learning: watching random topical videos, listening to random podcasts, reading an advanced-level book when you are still a beginner, and practicing complex codes are considered unstructured. The best option for anyone who wishes to get started with data science is to sign up for a structured training program.
The reason for our emphasis on the structured learning method is that it gives good shape to your learning process. You can measure your progress against strategies and go from a total beginner to an expert in a dedicated time frame with the right mix of consistency and commitment.
Choosing the right learning platform is another battle for beginners. There are thousands of online courses available, but the problem is to figure out which one will satisfy all your learning needs and give you a rewarding career straight after the course. One way to sort the tension is by analyzing the benefits and drawbacks as soon as possible. Don’t spend a lot of time in this process, it might eat away your interest, and you’ll probably never start learning.
Choose the right platform that best suits your learning style. Look for the meaningful edges of the course. It can be something like certifications, networks, expert mentors, practice platforms, offline availability of the content, and more.
Also, remember that everything comes with a price, just like your time and skill. So choose the course that you consider valuable for the money and effort spent. After signing up for the course, learn confidently and with complete perseverance. You got that!
If you are struggling to find the right learning path, check out GUVI’s Zen class – Data Science program to learn the in-depth topics of Data Science and practice real-life projects using gamified practice platforms. You could go from a complete beginner to a successful data scientist with this live-online course that will help you benefit from the globally recognized certifications and dedicated forum support.
Kickstart your Data Science journey by enrolling in GUVI’s Data Science Course where you will master technologies like MongoDB, Tableau, PowerBi, Pandas, etc., and build interesting real-life projects.
Alternatively, if you would like to explore Python through a Self-paced course, try GUVI’s Python certification course.
Wrapping up
Learning from your mistakes is more important than tracking your achievements. Keep yourself open to any suggestions and comments from experienced data scientists and Data experts. Follow these learning routines even after you land a job. However small, these precautions will keep you ahead in the right direction to becoming a successful Data scientist. Ensure you avoid these mistakes coming your way. Happy Learning!
FAQs
1. How do I start a data science career for beginners?
To begin a career in data science, start with understanding the field. If you’re a professional looking for upskilling options, sign up for a suitable online course to start by learning the fundamentals like mathematical concepts, probability, statistics, and more. Extract knowledge from webinars, podcasts, and social networks. Progress to the advanced level like Machine learning, Big data, data visualization, and storytelling.
2. Can a beginner do data science?
Yes, Beginners can undoubtedly get started with Data Science. With extensive knowledge of fundamentals, hands-on experience in real-life projects, and consistent learning, even a total beginner can transform into a pro in Data science. To build a career in data science, a beginner can sign up for a comprehensive course to master the relevant skills and become a certified data scientist.
3. Is data science hard for non-programmers?
No. With the help of modern-day tools and advanced algorithms, anyone can learn data science with little programming knowledge.
4. How to start a career in data science with no experience?
If you are a student with no experience, start learning the fundamentals of data science and build a strong foundation of the essentials. Build your knowledge by participating in webinars, podcasts, and social networks. Enhance your analytical and other technical skills by learning advanced concepts in Data Science through a comprehensive course. Get job-ready by earning certifications.
5. What is the fastest way to learn data science?
The quickest way to learn Data Science is by working on solving real-world business problems. A short-term online course that covers basic to advanced-level concepts will help you explore the field of data science in 3-6 months.
Did you enjoy this article?