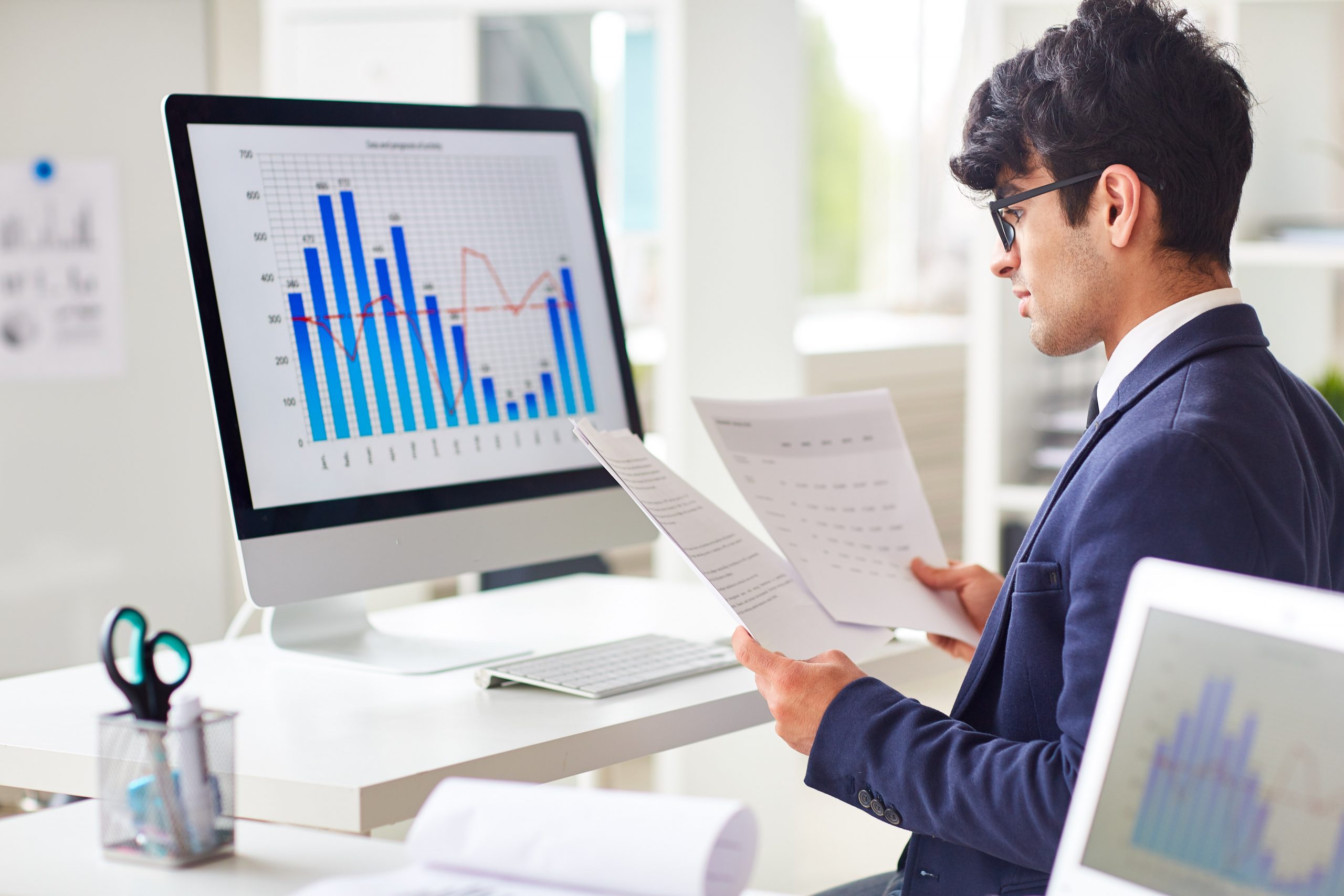
How to become a Data Scientist after Mechanical Engineering?
Apr 16, 2024 3 Min Read 3374 Views
(Last Updated)
Switching careers can sound quite intimidating especially when you’re a mechanical engineer by educational qualification, & willing to break into the Data Science industry as a Data Scientist. While dreaming of a data science career is a sign of forward thinking. Do you know how? According to ‘The Humans of Data Science’ report published by Michael Page India(a global recruitment firm), the demand for data science professionals is expected to increase globally by 11.5 million by 2026. Indeed, Data Science is a smart choice for making a promising career.
But, how do you get started in data science with mechanical engineering background? Before we jump into that, It’s also essential to know how data science is advantageous for mechanical engineers.
- Widened Career Scope
Although mechanical engineers have ample opportunities, a full-fledged data science professional will lead in the game of opportunities as they are most sought after in various fields like IT, E-commerce, FinTech, Healthcare, Retail, Travel & Tourism, Banking & Insurance, & so on.
- Lucrative Salary Packages
Ever heard of a data scientist who is struggling with a meager salary? Is this situation the same for Mechanical Engineers? Well, data science professionals are popular for their handsome salary packages. An entry-level data scientist earns a salary of ₹5 LPA & which goes up to ₹26 LPA for having better years of work experience with expertise.
Table of contents
- How to Switch from Mechanical Engineering to Data Scientist Job?
How to Switch from Mechanical Engineering to Data Scientist Job?
It gets easy-peasy when you focus on obtaining the below-mentioned skills for launching yourself into the data science future.
- Programming Skills
Programming has been recognized as the most important skill for data science professionals. A software background for a data science professional is like a solid backbone where they are a self-sufficient expert who does not need external resources to work with data. For most mechanical engineering graduates, MATLAB & Python are familiar programming languages. If mechanical engineers can learn related languages such as Python, SQL, Scala, Git, and R, then it becomes a cakewalk to transform into versatile data scientists, who build programs & algorithms to analyze data
- Data Skills are a Modern Asset
Though mechanical engineering is considered an evergreen field, the data science domain is booming with the increase in demand for data skills since companies that focus on growth use data to make business decisions.
The recruiters welcome people with various backgrounds who can help them analyze that data, & this is where mechanical engineers can jump into the data science domain with their cutting-edge data skills. Further, domain knowledge becomes essential as it helps the data science professional to understand the business & its products in terms of data.
Data scientists are expected to use core knowledge & machine learning algorithms for creating models. This helps them in high-value predictions that can arrive at better decisions and smart actions in real time without much human intervention.
Before we move into the next section, ensure you have a good grip on data science essentials like Python, MongoDB, Pandas, NumPy, Tableau & PowerBI Data Methods. If you are looking for a detailed course on Data Science, you can join GUVI’s Data Science Career Program with Placement Assistance. You’ll also learn about the trending tools and technologies and work on some real-time projects.
Additionally, if you want to explore Python through a self-paced course, try GUVI’s Python self-paced course.
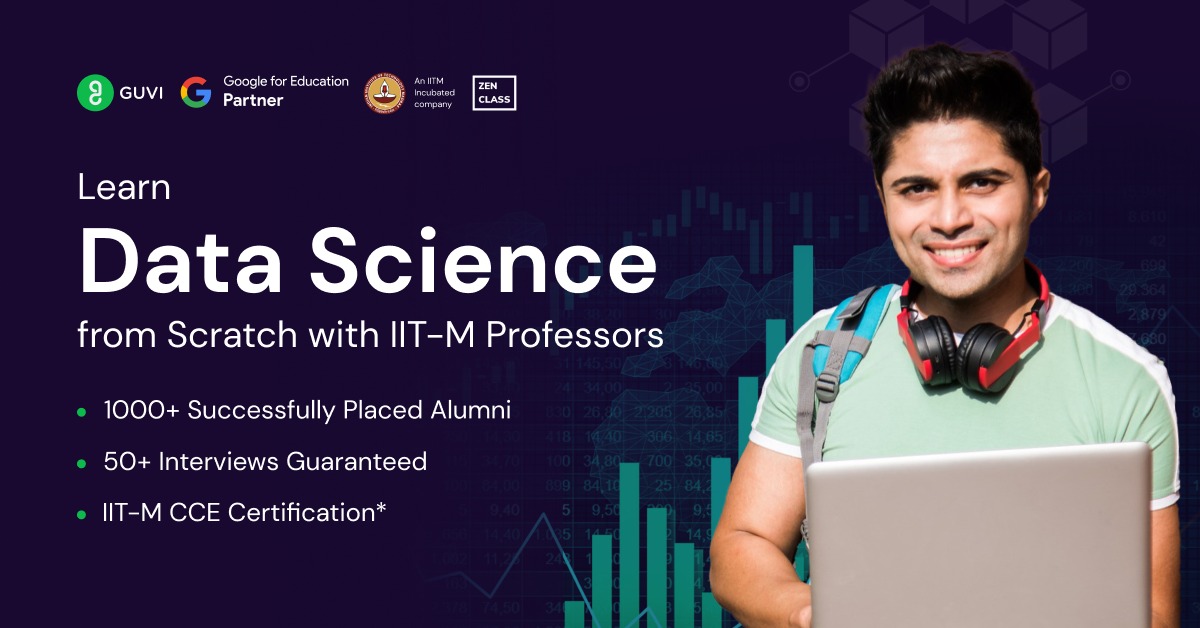
- Mathematics and statistics
Being a mechanical engineer will make you quite familiar with mathematics. While the level of application of maths could be different in data science, however, this familiarity helps a lot in machine learning algorithms and performing analyses. Also, discovering insights from data also requires math. And next, why statistics?
Data scientists are versatile professionals who work as researchers, programmers, business executives, etc. Statistics is a common subject that helps a data scientist perform multiple roles. Thus, a focus on statistics will enable data science professionals to gather, review, analyze, and draw conclusions from data. This also mobilizes them to apply quantified mathematical models to relevant variables.
- Data Visualization
Well, visualization is not restricted to just data visualization professionals, even data professionals like data analysts & data scientists use it to easily identify patterns, trends, and outliers in large data sets. This improves their work efficiency and helps them communicate their findings more effectively.
You know this, data visualization makes the data look more appealing & understandable to people than just rows filled with boring chunks of data.
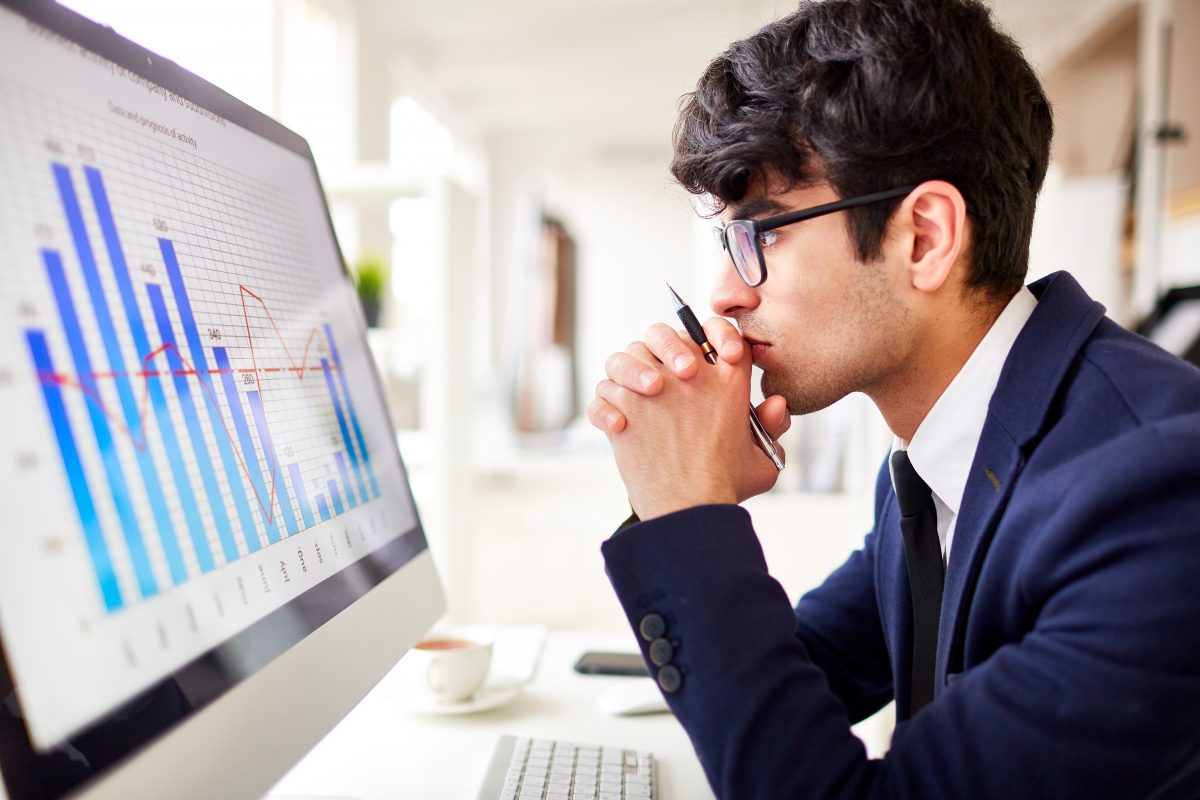
Next, an important realization is to understand that upskilling in the Data Science domain is not about just becoming a Data Scientist, but about getting eligible for other data roles like Data Analyst, Data Engineer, Business Analyst & So on. And pursuing these job roles depends on the niche & specific skills that you possess.
If you’re wondering what is the difference between these job roles, then this table might help you.
Data Scientist | Data Analyst | Data Engineer | Business Analyst |
Uses Mathematics, Statistics, Machine Learning Models, etc & makes predictions through data & finds solutions for key problems in businesses. | Brings out the meaning of the pulled data & conveying it to the company for making better business decisions. | A disguised software engineer with data skills, who builds & optimizes the systems with a perfect environment for data analysts & data scientists to store, pull & work on data. | Focuses on customer-related data with the product(s) & analyses the cross-departmental progress in terms of data. |
Tools & Technologies: Python, R, Machine Learning, PowerBI, NLP, etc. | Tools & Technologies: SQL, SAS, Microsoft Excel, Tableau, etc. | Tools & Technologies: Python, PySpark, SQL, Matplotlib, AWS: S3, EC2, Lambda, etc. | Tools & Technologies: Excel, SQL, Google Analytics, PowerBI, Tableau, etc. |
Kickstart your Data Science journey by enrolling in GUVI’s Data Science Career Program where you will master technologies like MongoDB, Tableau, PowerBI, Pandas, etc., and build interesting real-life projects.
Alternatively, if you would like to explore Python through a Self-paced course, try GUVI’s Python Self-Paced certification course.
Did you enjoy this article?